If the findings were at the forefront of science, many of the issues discussed in this article would have been accepted practice for decades. This is particularly evident when we look back to 1954, when US psychology professor Paul Meehl published his book "Clinical vs. Statistical Prediction". 1] It was already clear to him at that time that models clearly outperform experts in many areas. In his book, he described how formal, systematic methods offer a better way to assess the future behavior of a patient than the expert opinion of the treating psychiatrist or psychologist. Based on his research, he came to the conclusion that this also applies to diagnoses and treatment recommendations. The title of the last chapter in his book says it all: "A Final Word: Unavoidability of Statistics". In it, he called on his colleagues to open themselves to systematic analysis by objectively considering the following question in their daily work: "Am I doing better than I could do by flipping pennies? [2]
Of course this assessment was controversial at the time. For Meehl argued that the experts made more mistakes in their subjective, experience-based assessments than a mechanical tool that combines actual clinical data and derives a prognosis from it. According to Meehl, the experts might, for example, be subject to distorting behavioural effects by (unconsciously) searching for confirming information for ready-made assessments or by neglecting contradictory information. In addition, he pointed out possible overconfidence and possibly popular, empirically untenable, anecdotal observations. These "insinuations" were not well received by the respected experts at all and led to decades of debate.
More than 20 years after the publication of his book, Meehl summed up his unchanged assessment in an essay: "There is no controversy in social science that shows such a large body of qualitatively diverse studies coming out so uniformly ... as this one." [3]
Models outperform experts.
One could say that Professor Meehl was an early advocate of algo, albeit in a completely different field than the stock market. However, he was ahead of his time: Throughout his career, Meehl had to fight for modern methods to prevail and his recommendation to deviate as rarely as possible from systematically/statistically determined forecasts was accepted. As is to be expected from today's perspective, later studies proved him right: mechanical methods achieved better forecasts than classical, clinical expert assessments, as confirmed by a comprehensive meta-study:
"[...] mechanical predictions of human behaviors are equal or superior to clinical prediction methods for a wide range of circumstances." [4]
Experts vs. systematics
The author of the 2014 paper "Are You Trying Too Hard?", Wesley Gray, is also an advocate of systematic decision making. [5] His central argument is that simple, quantitative models with a limited number of parameters achieve better results than discretionary decisions by experts. Nevertheless, expert judgements are still in demand. According to Grey, this is due to three wrong assumptions that we make (unconsciously):
qualitative information increases the accuracy of the forecast
more information increases the accuracy of the forecast
experience and intuition increase the accuracy of the forecast
Indeed, these assumptions are not empirically tenable. We are misled by the feeling that our own efforts (or those of the experts) should be worthwhile to lead to good decisions on the stock market. However, in the long run, the information benefits of discretionary decisions - which may initially actually exist - are overcompensated by the costs caused by distorted perception and behavioral errors. Therefore, developing algorithms and entire, self-contained trading systems can be an astonishingly good solution, instead of always looking for new excuses and explanations for recurring human errors.
Checkershadow Illusion
That human errors can happen almost automatically on the basis of perceptual effects is shown in the following graphic, which is also known as the "checker shadow illusion": [6]
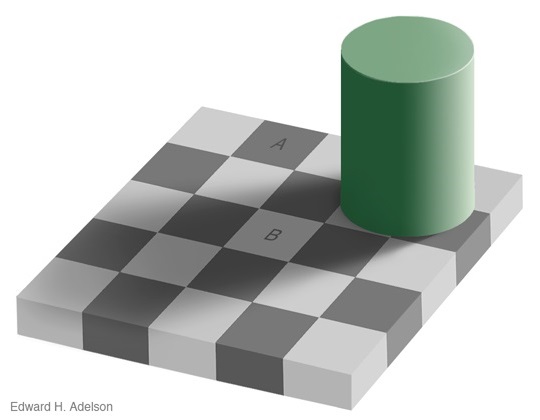
Which area is darker, A or B?
Source: Adelson, Edward H. (1995), Checkershadow Illusion, Massachusetts Institute of Technology
The human brain automatically estimates area A to be darker than area B. No expert in the world who does not already know this graph would claim otherwise. And yet we are deceived. Because if a computer examines the RGB color values of the two surfaces completely mechanically, the result is 100 percent identical: 120, 120, 120. The sober conclusion of the computer, which is not subject to any perceptual effects, is therefore: No difference. Both surfaces are exactly identical, i.e. equally light or dark. And the computer is (naturally) absolutely right.
However, our brain proceeds differently to assess the grey tones. Here, it is primarily a matter of using our visual perception to correctly assess the real world in order to survive in it - and experience values such as the influence of a shadow and the adjustment of the perceived brightness interpreted into it play a decisive role here. This process takes place unconsciously so that we can hardly believe that our eyes deceive us - but they do, demonstrably. If you don't believe this, print the graphic, cut the fields into pieces and place them next to each other. You will see that the greys are indeed identical. Alternatively, it is of course much easier to do this with a computer image editing program. Professor Adelson also did this work for us 25 years ago, as the following graphic shows [7]
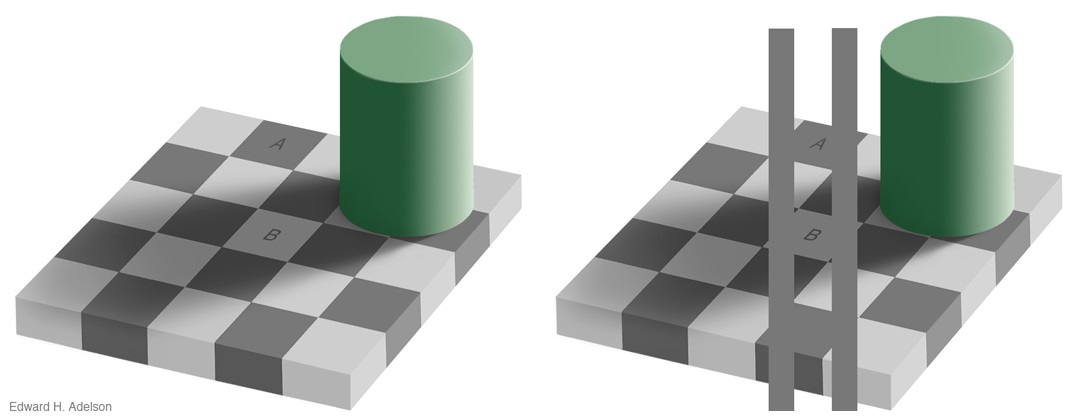
Indeed, both surfaces are likely light resp. dark.
Source: Adelson, Edward H. (1995), Checkershadow Illusion, Massachusetts Institute of Technology
Wesley Gray writes in his paper that experimental psychology has clearly shown one thing: Humans are not able to reliably distinguish between information that actually increases the accuracy of a prognosis and information that is ultimately completely unnecessary (or even counterproductive) but - wrongly - according to subjective assessment allows for an apparent improvement. Without direct proof that discretionary decisions by experts are actually better, however, especially on the stock market, all that often remains is the "guru story" about a certain person who (perhaps) was successful in the past - which is obviously not a reliable basis for achieving above-average results in the long term.
Algorithmic decisions
Daniel Kahnemann writes in the summary to his book "Thinking, Fast and Slow" that it is important to have a mechanical algorithm to compensate for human error. In his opinion, algorithmic approaches have the following advantages: [8]
Avoiding the bias to use immediately available information because of the need to obtain the inputs relevant to the algorithm
Avoidance of the tendency to rely on subjective probability weightings, as the algorithm works with predefined formulas
Avoiding the construction of an apparently conclusive mental "story" by using the algorithm to produce an objective result in the form of a number
On the basis of his considerations, Kahneman comes to a clear conclusion regarding the importance of expert assessments in the financial markets:
"[...] financial experts on the stock market whose performances are disappointingly weak when checked against the future, [are] in fact seldom more efficient than the random advice a monkey could have supplied throwing darts on a board. [...] an individual player, looking for trends, is in a position no better than the hapless gambler by the casino. "The house always has the upper hand."“[8]
Combination of human and algorithm?
From the perspective of scientific research, (simple) quantitative models achieve significantly better results than expert assessments in most areas. Therefore, it might be useful to provide human decision makers with the results of the models as input to possibly achieve even better combined results. However, according to an analysis by capital market analyst James Montier, this is precisely what is not working: He concludes that even then the experts still achieved worse results than the pure model [9]. According to Montier, this illustrates a crucial point: "Quantitative models represent an upper limit of possible outcomes, from which, in the case of human influence, a corresponding deduction must be made - and not, conversely, a lower limit to which discretionary decisions represent additional added value. As a reason for this, he cites the fact that we overweight our own decisions when combined with the statements of the model.
In his analysis, Montier describes himself as the best example of precisely this scenario. He published a model for tactical asset allocation based on a combination of valuation and momentum. Initially, the model produced signals that corresponded to his personal, at the time bearish, basic assessment. But then the model unexpectedly produced bullish signals, which he did not implement because he thought his own assessment was better - despite successful backtests that showed that the model worked. In this way, he managed to underperform his model for about 18 months.
Why are there so few quants?
If quantitative models are so good and actually represent an upper limit to the results that are possible in the long term, why are there so few providers who work exclusively with them? Shouldn't these models, due to their above-average results over time in the context of evolutionary dynamics, be able to prevail in the markets?
Montier's paper also provides some explanations for this: [9]
Overconfidence: this effect leads market participants to believe that they can supplement the model or improve it by omitting signals in certain situations
Right to exist: A large proportion of employees in (institutional) business would lose their jobs if only quantitative models were used
Unwillingness to change: Large companies that are successful on the market with processes that have been tried and tested for decades would have to throw most things overboard
Sales arguments: quantitative models are harder to sell because they are often interpreted as a black box or the process is degraded in comparison to human-operated products ("the algorithm does all the work")
All in all, these points represent a high hurdle for quantitative models to achieve a sustainable breakthrough. Incidentally, this does not only apply to the financial industry. Kahneman also describes similar obstacles for other areas:
"But of course, clinicians, political pundits, and financial advisors have more than a vested interest in keeping up the illusion." [8]