The perfect sniper
How hard is it to hit the exact center of a target drawn on a wall with a long-range shot? It might be about as difficult as finding a trading strategy that produces above-average returns in the markets.
But you can also make it easier on yourself with the target by shooting into the wall first and then drawing the mark around the shot. This may sound ridiculous, but it is regularly practiced in capital market research to produce bull's-eyes. US economics professor Gary Smith aptly describes this approach as the "Texas Sharpshooter Fallacy." [1]
Something extremely improbable is not improbable at all if it has already happened.
The example is of course exaggerated, but nevertheless makes an important point: examining common characteristics of companies that have already been selected as successful in advance is not particularly meaningful. According to Smith, a scientific method should be used instead:
- Selecting in advance the characteristics to be studied and logically justifying why they predict subsequent success.
- Selecting in advance companies that have these characteristics and those that do not.
- Analysis of success in the years to come based on pre-determined criteria
False discoveries
If you search for successful trading strategies in this way, you will find that there are very few of them. The reason for this is the strong competition on the markets, which leads to a high degree of efficiency. If a profitable strategy is discovered, it usually only works for a limited time. At the same time, the danger of making false discoveries is very high. This is because, unlike in the natural sciences, statistical findings in the markets can hardly be verified by controlled experiments.
One example is alternative asset classes such as wine or art, which have allegedly performed better than the stock market. If one looks more closely, the data often comes from asset managers who are involved in these assets, which represents a clear conflict of interest. The following chart from Factor Research shows which data sources one should be cautious about when assessing reliability. [2]
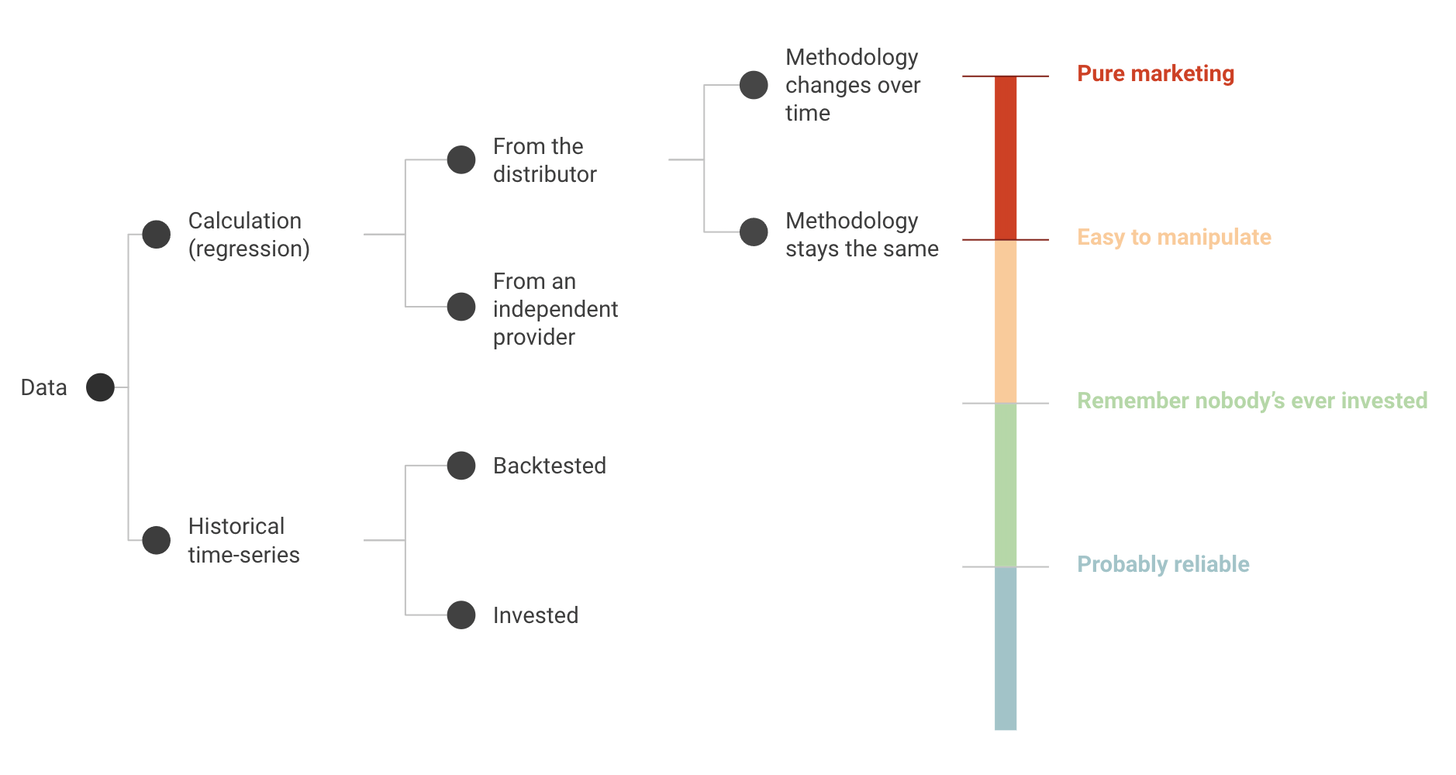
Source: Factor Research [2]
At the same time, many investors are not aware that high standards are necessary to really trust a particular approach. So, they often buy what looks good - under the assumption that the developers already know what they are doing.
In reality, however, the financial industry is dominated by over-optimised backtests that sooner or later lead to bitter disappointments in their implementation. But how can it be that such an unprofessional approach has become common practice?
The problem with backtests
One explanation is the way back-testing of investment strategies is done. Developers usually use historical market data, which are computer-assisted and analysed for a multitude of criteria, weightings and combinations. From this, an optimal design can be determined and a potential return can be indicated that can be expected based on the simulations.
As the paper "Finance Is Not Excused" describes, this results in over-optimised back-calculations that are not meaningful for the future. [3] The reason for this is that far too many variants are tried out in relation to the amount of data available, and thus random patterns are (unconsciously) taken into account as relevant. The result: seemingly good strategies disappoint in practical implementation, the most "honest" of all out-of-sample tests.

Source: Huang, S. / Song, Y. / Xiang, H. (2020), The Smart Beta Mirage, p. 5. [4]
The chart above shows two smart beta indices relative to the market as an example. Both reflect the same factor - the grey curve in the original variant created in 1997 and the red curve in the variant improved in 2014. While the original variant did not outperform, the new version looked much better in retrospect. Then an ETF was launched, which of course tracked the new index. But its better performance probably only occurred in the backtest. The fund underperformed right from the start. [4]
The high computing capacity of modern computers has further exacerbated the problem. Today, millions or billions of parameter combinations can easily be examined. And if the developers then find "significant" statistical patterns, it is not difficult to draw a suitable explanation around them - similar to what the clever sniper did at the beginning. Unfortunately, according to the study, this also seems to be the rule rather than the exception in the development of investment strategies.