The five "evils"
First, de Prado summarises the five major problems that quants face. There is no definitive solution to these, nor can one avoid them. Instead, one must learn to cope with them in the best possible way.
1) Barriers to experimentation:
A fundamental principle of science is empirical falsification. In the natural sciences, this is made possible by controlled experiments. In the financial industry, however, this is rarely possible. This is because we cannot repeat the flash crash of 2010, for example, and in doing so fade out the influence of certain groups of market participants, because they interact. Therefore, it is very difficult to determine clear connections between cause and effect.
2) Non-stationarity of financial markets:
On the one hand, there are structural breaks caused by regulatory changes or unforeseeable events (black swans). On the other, parameters that affect the basic process for generating price data can be subject to drift, for example due to competition between companies. So even if correlations between cause and effect are established, their intensity can change significantly over time.
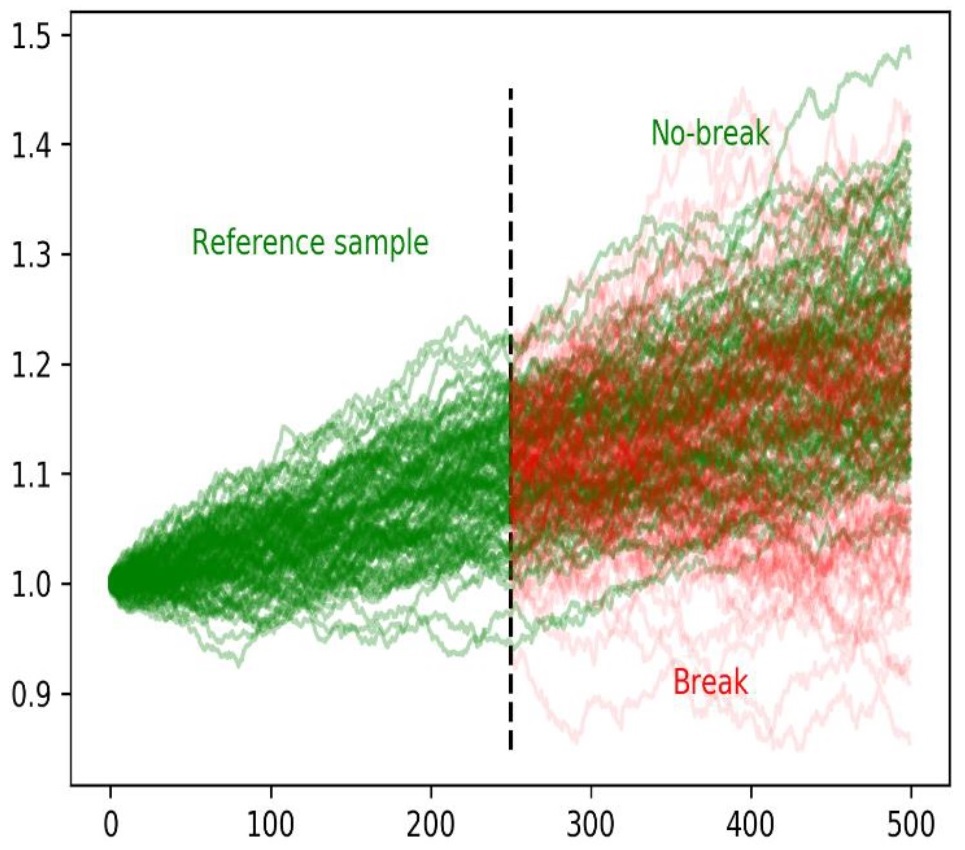
In this example, a structural break occurs after 250 observations with a probability of 50 percent. Subsequently, either the green or the red course result. The problem is to detect the break as quickly as possible, but also as reliably as possible.
Source: de Prado, M. L. (2021), Escaping The Sisyphean Trap: How Quants Can Achieve Their Full Potential, p. 9.
3) Rigorous competition (zero-sum game):
Fierce competition among market participants worsens the ratio of actual signals to mere noise. The probability of finding profitable strategies is therefore extremely low. And if one does discover alpha, it is in most cases only scalable to a limited extent and/or quickly regressive. This is also evident in the fact that the publication of research findings affects their future validity.

Suppose that the probability of a tested strategy to be profitable is one per cent. With the usual threshold of five per cent used in significance tests and an (optimistic) statistical power of 80 per cent, one would expect to make a total of 58 discoveries in 1000 trials. Of these, eight would be true positives and 50 false positives. In other words, about 86 per cent would be false!
Source: de Prado, M. L. (2021), Escaping The Sisyphean Trap: How Quants Can Achieve Their Full Potential, p. 10
4) Complexity of the system:
Econometrics was developed to model relatively simple processes. It assumes that one knows the predictive variables and the functional form including all interaction effects. If this is not the case or if the function is incorrect, true predictive variables can be rejected as false negatives and false ones accepted as false positives. The (alleged) knowledge of the function should therefore not be assumed. For this may in fact be highly dimensional - or even impossible to determine.
5) Small samples:
Financial data sets are often short time series with few variables and high mutual, as well as, serial dependencies. Therefore, inferences are often drawn from small samples, which involves heavily overfitting of training and test data. This means that statistical tests at the standard confidence levels used have a low significance, a high proportion of false-negative results as well as many false discoveries. As an example of this, capital market professional Mark Rzepczynski cites the countless business cycle analyses that are largely based on only eight such cycles since 1970. How significant can these studies really be? [2]
Problematic structures
Traditional asset managers often rely on small, independent quant teams that do not collaborate but compete for the allocation of capital. The goal here is to achieve diversification through competition. However, this structure suffers from several problems:
• lack of research depth, as teams are mostly made up of generalists
• knowledge that cannot be reused if the intellectual property belongs to the teams
• no mechanisms for self-correction due to lack of collaboration
• incentives for false positives as a result of competition.
The conclusion is that classical silo structures or platforms for scientific talent are not optimal as they limit the potential of quant teams.
Sisyphean work
As a result, such teams may end up performing repetitive, pointless tasks such as backtesting, leading to an endless cycle of false discoveries. A good comparison to this is Sisyphus' work, which comes from a legend in Greek mythology. This refers to work that is not only fruitless and difficult, but will also never be done, as one has to start all over again. [3]
According to de Prado, to best deal with the five evils of financial market research, co-specialised teams are needed. A division of labour is essential to implement the control mechanisms anchored in scientific methods, such as peer review and empirical falsification. So what is his proposed solution?